Using Al can be like setting off an expedition into uncharted land. Even with potential advantages such as process automation and intelligence, the journey can be extremely challenging. Even the most promising Al projects run the danger of drifting without a clear plan or the appropriate instruments in place.
Al projects call for more than just ambition; they also entail rigorous alignment with corporate goals, a comprehensive readiness assessment, and a strong emphasis on ethical, data-driven decisions to assure success. Businesses must understand that Al is not a universal solution, and they can only fully realize its potential by meticulously tailoring a strategy to their specific needs.
Furthermore, gathering, governance, and data usage are crucial for Al’s ethical acceptance. Building Al models with real value depends mostly on ensuring data quality and reducing risks.
1. Strategy and Business Alignment
The success of any artificial intelligence project mostly depends on how well it complements the main corporate goals of the company. Whether it’s to simplify processes, boost customer experience, or sharpen decision-making, it’s imperative to find AI initiatives with real worth. First, engaging people from other departments helps one better understand their problems. For instance, artificial intelligence can assist companies in automating various P2P process steps, such as the responsibilities of a requestor, approver, buyer, receiver, and invoice approver, as well as automating mundane and time-consuming downstream processes, thereby maximizing operational efficiency in supply chain management.
Consider a retail business using AI to predict inventory shortages. Here, the integration of AI with their ERP system ensures that predictions not only inform purchasing decisions but also maintain supply chain continuity. By linking responsible AI outcomes with actionable business insights, companies can prevent inventory shortages, thereby improving customer satisfaction and revenue. This kind of strategic alignment—where AI output directly impacts critical business functions, ensures that the initiative is both valuable and scalable.
2. Feasibility and Readiness Assessment
Once you’ve identified potential AI use cases, the next step is to assess the feasibility of those projects. This involves evaluating whether the organization has the necessary resources, talent, and infrastructure to support AI deployment. For instance, does the organization possess sufficient computing power? Does it have access to high-quality, well-labeled data? Is there in-house expertise in AI or will external vendors be required?
An unsaid rule of AI, start small. Companies that initiate pilot projects or Proof of Concept (PoC) testing before rolling out ethical AI solutions across their operations often have better success rates. This approach not only validates the value of the AI initiative but also helps create internal momentum, making larger-scale AI adoption smoother.
3. Dealing with Data Silos and Data Governance
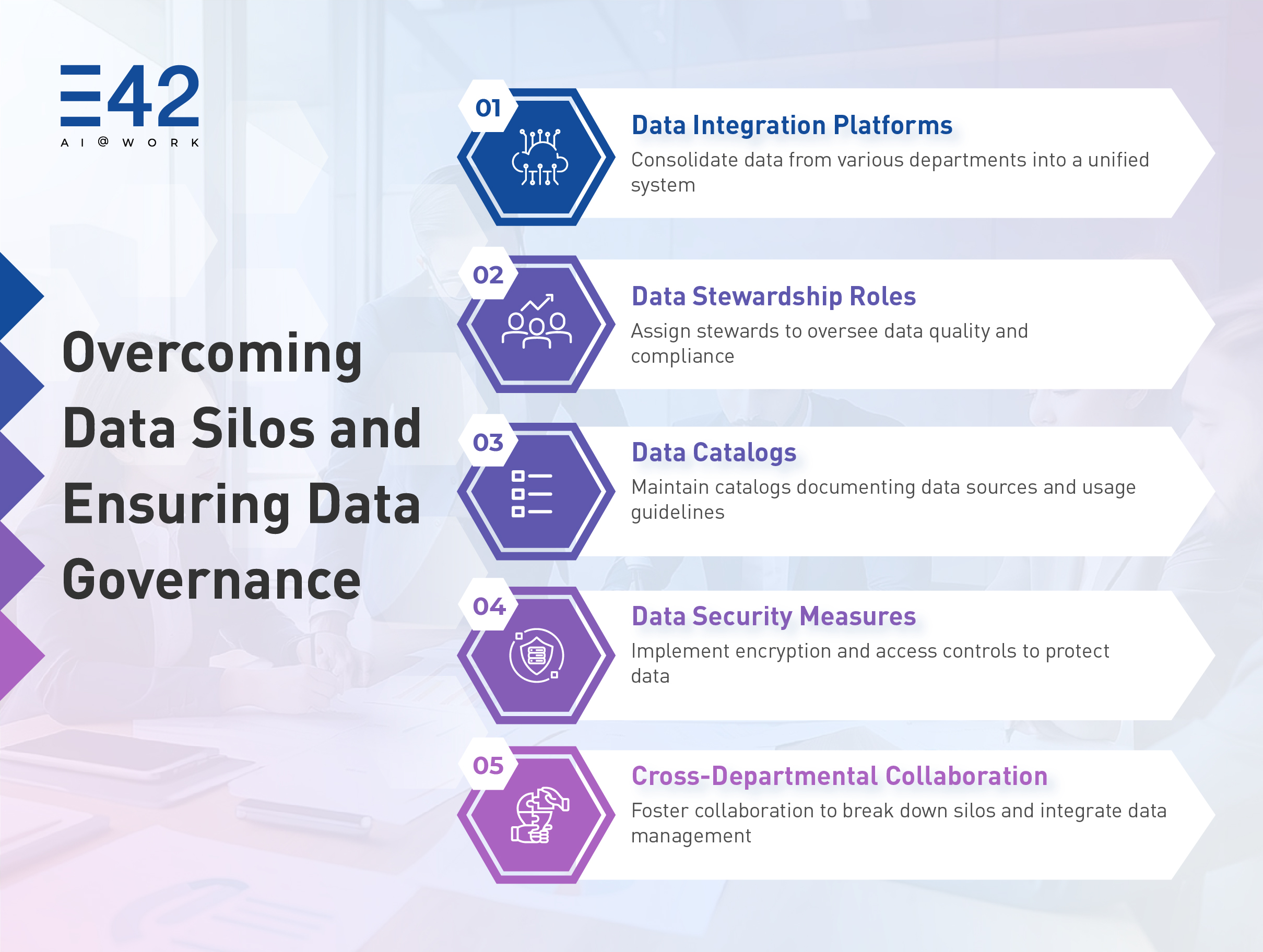
One of the most persistent barriers to AI success is the presence of data silos within organizations. These silos are created when departments, such as finance, marketing, or operations, store data in isolation, making it difficult to access and leverage across the organization. This fragmented approach hinders comprehensive AI implementation, as AI models rely on diverse datasets to generate accurate insights.
To address this, many businesses are opting for on-premises Large Language Models (LLMs) and omni-channel integrations that can pull data from different sources and centralize it. On-premises LLMs offer the advantage of better control over data security and governance, a key concern for industries handling sensitive information, such as BSFI or healthcare. Unlike cloud-based solutions, on-premises models allow organizations to process data without sending it offsite, which can help ease concerns related to data privacy and compliance.
Data governance also plays a critical role. For AI guardrails to be effective, organizations must ensure that the data they feed into their models is accurate, relevant, and representative of the problem at hand. Establishing robust data governance practices—including creating data pipelines, standardizing data across departments, and ensuring the vendor meets the regulatory compliance (e.g., SOC 2 Type II, ISO 27001:2013, GDPR, HIPAA)—is crucial for AI success.
4. From Rule-Based to Learning-Based Systems: Breaking Tradition
Traditional rule-based systems in areas like finance and operations are rapidly giving way to responsible AI-driven, learning-based systems. While rule-based systems can automate processes based on pre-defined rules, they are limited by their rigidity. For instance, a rule-based system might automate invoice approval based on predefined thresholds (e.g., invoices below $10,000 are automatically approved). However, it cannot learn or adapt to new patterns, such as changes in vendor behavior or unusual spending patterns.
Cognitive Process Automation (CPA), on the other hand, thrives in such environments. AI-driven Accounts Payable (AP) automation goes beyond rule-based systems by learning from historical data to improve over time. It can adapt to varying invoice formats, even handling complex cases such as handwritten invoices or deviations in data. This adaptability significantly enhances operational efficiency, reduces human errors, and streamlines processes.
A practical example is an AI AP Executive built on E42 that can seamlessly integrate with an organization’s ERP system. By leveraging Intelligent Document Processing (IDP), the AI can handle everything from data ingestion, validation of invoice data with PO, GRN tables fetched from the ERP to invoice approval and posting the MIRO entries into the ERP for further downstream process, cutting down processing time and reducing errors. Traditional rule-based methods are being outpaced by AI’s ability to learn and evolve.
5. Data Ingestion Time: Accelerating the Path to Actionable Insights
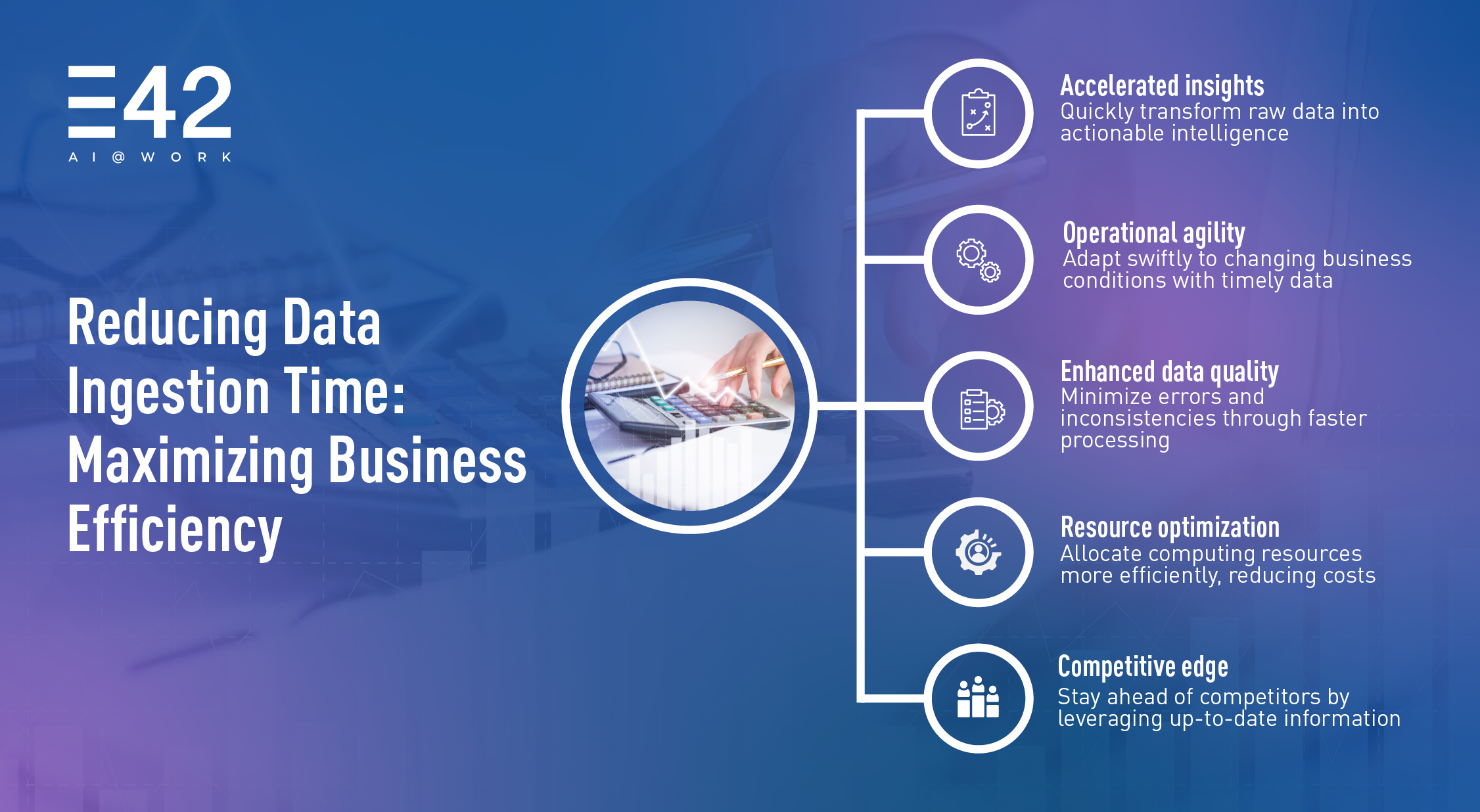
The time it takes to ingest and process data is a critical guardrail for AI projects, particularly for time-sensitive industries like BSFI and healthcare. Data ingestion—the process of importing, processing, and storing data for AI models to use—can be a bottleneck if not optimized. AI implementations that offer faster data ingestion times allow for quicker insights and more agile decision-making. This is achieved through Kubernetes and containerization help improving scalability, reduce latency, resiliency, and enhanced data quality.
AI solutions that streamline data ingestion, such as automated data pipelines, can drastically reduce the time it takes to prepare data for model training and analysis. For example, an ethical AI system deployed in a hospital setting can ingest patient data from electronic health records, process it in real time, and provide actionable insights to doctors, helping them make faster and more informed decisions. In the context of business, faster data ingestion helps companies identify trends more quickly, leading to timely, data-driven actions.
6. Regulatory and Ethical Compliance
With the rise of AI, regulatory and ethical compliance has become a pressing concern. AI algorithms must be transparent, accountable, and free from bias. The European Union’s AI Act and other global regulations are setting new standards for how AI models should be governed.
Following extensive consultation with CIOs worldwide, Gartner proposed a Trust, Risk, and Security Management (TRiSM) framework. This framework guarantees the governance of AI models, their trustworthiness, fairness, reliability, robustness, efficacy, and data protection. This includes solutions and techniques for model interpretability and explainability, AI data protection, ethical AI, guardrails in AI, model operations, and adversarial attack resistance.
Organizations implementing AI must build systems that not only comply with current regulations but also anticipate future ones. This involves creating transparent AI systems that allow stakeholders to understand how decisions are made and ensuring that these systems are audited regularly. Ethical considerations should also include efforts to mitigate bias in algorithms. For example, in the financial sector, AI models should ensure that credit-scoring systems don’t disproportionately disadvantage certain demographic groups.
7. AI Co-Workers: Enhancing Productivity Through Collaboration
The idea of AI co-workers is no longer a futuristic concept; it’s a reality in many businesses today. AI systems are now acting as collaborative partners in various sectors, working alongside human employees to augment their capabilities. In customer service, AI chatbots and virtual assistants are automating routine tasks, allowing human agents to focus on more complex issues. In manufacturing, AI-driven robots are working alongside factory workers to boost productivity and reduce errors.
AI co-workers are particularly impactful in areas like Accounts Payable, P2P, O2C where they handle repetitive tasks, freeing employees from chores to concentrate on core activities. By integrating AI co-workers into the workforce, companies can create a more agile and efficient organization, enabling faster decision-making and higher productivity.
8. Risk Management and Mitigation
Risk management is a critical component of any AI project. The implementation of AI carries risks, such as technical failures, cybersecurity threats, or operational disruptions. Organizations must create risk mitigation strategies, including implementing cybersecurity measures, conducting routine audits of AI systems, and developing contingency plans in case of system failures.
For instance, a financial institution deploying responsible AI for fraud detection must ensure that its AI system is robust against cyberattacks. This involves collaborating with IT security teams to safeguard AI models from being compromised, as well as continually monitoring the system to detect any vulnerabilities.
9. Talent Development and Upskilling
Lastly, no AI initiative can succeed without the right talent. Organizations must invest in upskilling their workforce to meet the demands of AI. This includes training programs in machine learning, data science, and AI ethics. It also involves fostering a culture of continuous learning and innovation, encouraging employees to stay ahead of the curve in an ever-evolving technological landscape.
A practical approach to talent development is creating ethical AI centers of excellence within the organization. These centers can serve as hubs for training employees, testing new AI solutions, and developing best practices for AI implementation.
Conclusion: Leveraging AI for a Competitive Advantage
In conclusion, AI-powered project identification and implementation require a well-structured approach that encompasses business alignment, data governance, ethical considerations, and a focus on human-AI collaboration. By setting clear guardrails and adhering to best practices, organizations can unlock the full potential of AI to achieve their strategic objectives.
From on-premises LLMs to AI co-workers, the future of AI is not just about automation but about creating more intelligent, collaborative, and adaptive systems that drive sustainable growth and innovation in an increasingly complex digital landscape. By investing in AI today, businesses are not only enhancing their operational capabilities but also paving the way for long-term success in an AI-first world.
To discover how to effectively leverage AI for your enterprise without compromising on security, write to us at in******@e4*.ai .