“The real problem is not whether machines think but whether men do.”
– B.F. Skinner
In a world where machines don’t just follow orders but think for themselves, make decisions, and continuously learn from their surroundings, agentic AI is no longer a distant dream. This shift challenges us to rethink our roles and responsibilities, emphasizing the importance of human creativity and strategic thinking in the age of intelligent machines. In the context of enterprise automation, agentic AI is set to revolutionize business operations by embedding self-directed intelligence into everyday systems.
Agentic AI represents a significant leap in the development of AI agents, enabling them to function autonomously, make complex decisions, and continuously learn from their experiences without requiring ongoing human supervision. This evolution is reshaping the capabilities and roles of AI agents in various domains. In this blog post, we’ll explore the technical intricacies of agentic AI, its journey into the business world, the immense potential it holds, and the hurdles it must overcome.
Understanding Agentic AI
Agentic AI refers to artificial intelligence systems that exhibit a high degree of autonomy, allowing them to operate independently and make decisions without continuous human oversight. Unlike traditional AI models that require explicit instructions for every task, agentic AI is designed to set its own goals, adapt to new information, and evolve its strategies over time. This type of AI mimics aspects of human cognition and decision-making, enabling it to manage complex tasks and environments effectively.
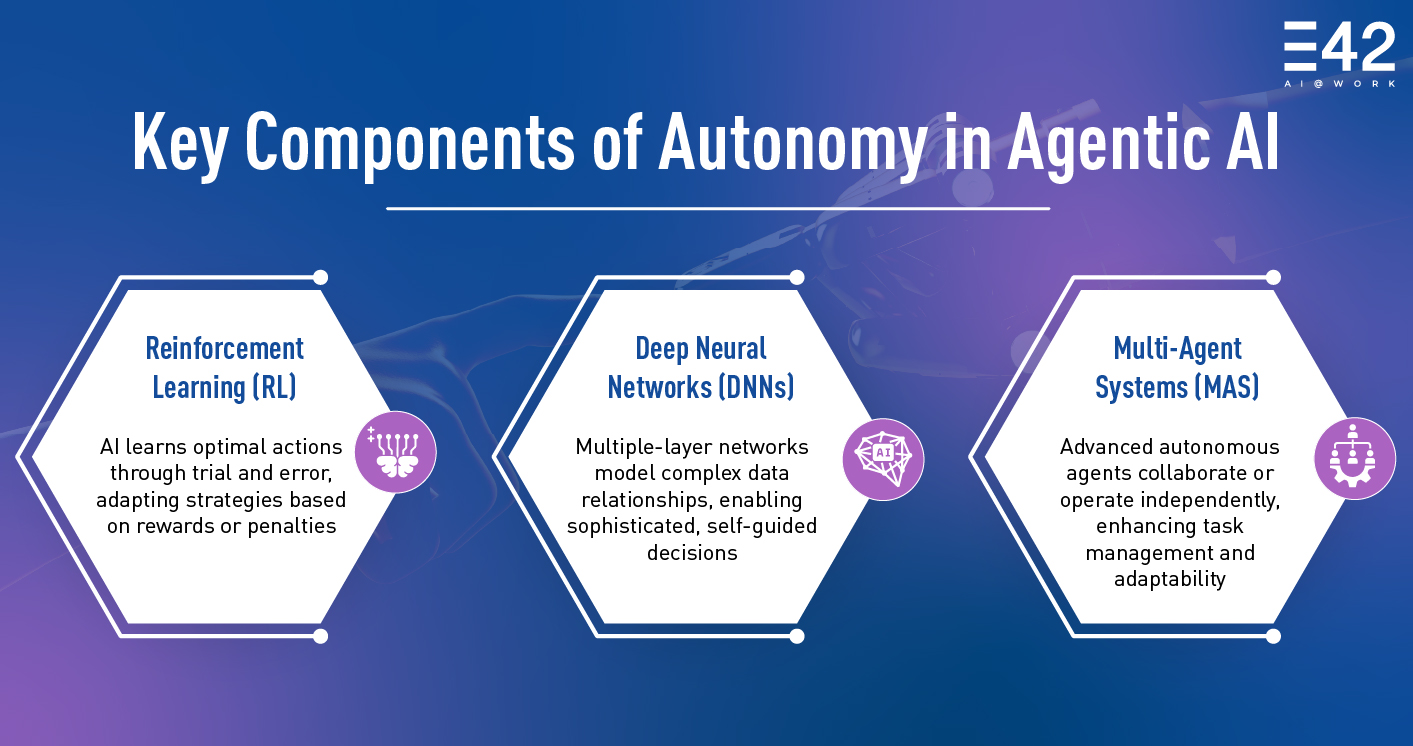
Autonomy
Autonomy in agentic AI is achieved through advanced algorithms and architectures that allow the system to function independently. Key components include:
- Reinforcement Learning (RL): RL algorithms enable AI systems to learn optimal actions through trial and error, receiving rewards or penalties based on their actions. This allows them to adapt their strategies and improve their performance over time.
- Deep Neural Networks (DNNs): DNNs, especially those with multiple layers, can model complex relationships in data, enabling AI systems to make sophisticated decisions and predictions without explicit programming for every scenario.
- Multi-Agent Systems (MAS): MAS involves multiple AI agents working together or independently, these intelligent autonomous systems share information and coordinate actions. This setup enhances the system’s ability to manage complex tasks and adapt to dynamic environments.
Self-Direction
Agentic AI systems can set and pursue their own goals through mechanisms such as:
- Goal-Setting Algorithms: These algorithms allow AI systems to define objectives based on their environment and prior experiences. For instance, an AI managing a data center might set goals to optimize energy consumption or improve system uptime.
- Adaptive Learning: Techniques like transfer learning enable AI systems to apply knowledge gained from one task to new, related tasks. This adaptability allows agentic AI to improve its performance and handle novel situations effectively.
Decision-Making
Decision-making frameworks for agentic AI include:
- Decision Trees and Graphs: These models help AI systems evaluate various decision paths and choose the most optimal one based on current information and predicted outcomes
- Bayesian Networks: Bayesian networks provide a probabilistic framework for decision-making, allowing AI systems to make informed choices based on uncertainty and incomplete information
- Temporal Logic: This approach involves decision-making based on temporal sequences, making it useful for applications that require planning and forecasting over time
Agentic AI vs. Generative AI: Distinct Roles and Synergistic Benefits
Agentic AI and generative AI are distinct yet complementary aspects of artificial intelligence, each playing a unique role. Agentic AI focuses on autonomy and real-time decision-making, driven by algorithms like reinforcement learning and adaptive control systems. This allows systems to manage complex tasks and adapt to changing environments with minimal human intervention. In contrast, generative AI is all about creating new data or content based on patterns learned from existing datasets, utilizing techniques such as Generative Adversarial Networks (GANs) and transformers. While agentic AI excels at decision-making and managing processes, generative AI is adept at producing realistic text, images, and audio.
When combined, these two types of AI can create powerful synergies. For instance, AI agents/AI co-workers might use generative AI to enhance its decision-making capabilities by generating predictive insights or simulating various scenarios. Conversely, generative AI can leverage agentic AI’s autonomous Intelligence to optimize and refine its content generation processes. This interplay enables organizations to benefit from both robust, autonomous operations and creative, data-driven content production, driving innovation and efficiency across various applications.
Benefits of Incorporating Agentic AI in Enterprises
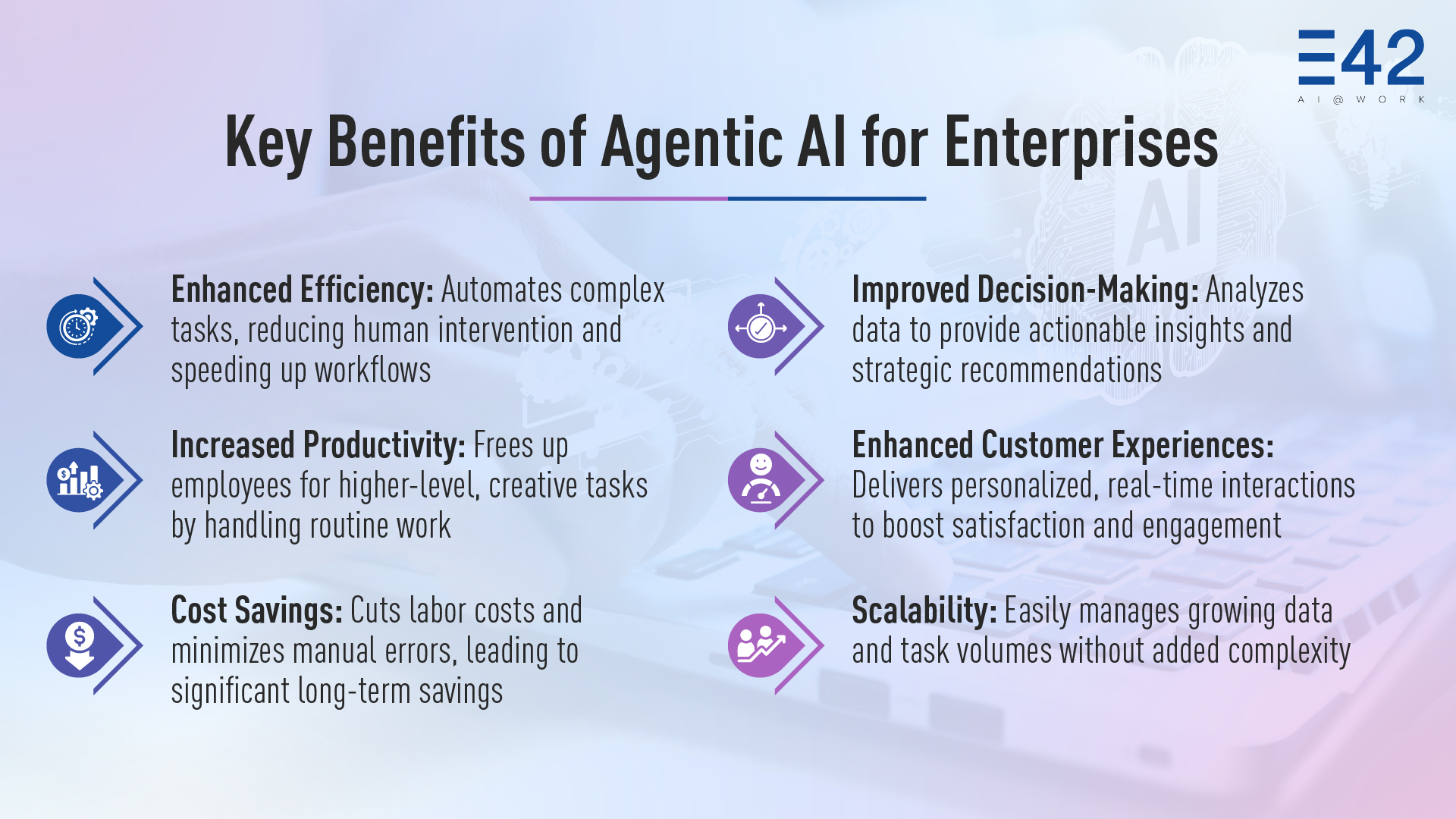
Incorporating agentic AI into enterprises brings a range of transformative benefits that can revolutionize operations and enhance overall performance:
- Enhanced Efficiency: Agentic AI automates complex and repetitive tasks, reducing the need for human intervention and accelerating operational workflows. This results in faster processing times and streamlines business operations.
- Improved Decision-Making: With their capability to analyze vast amounts of data and recognize patterns, AI agents provide actionable insights and data-driven recommendations. This enhances decision-making processes, allowing businesses to make more informed and strategic choices.
- Increased Productivity: By offloading routine and mundane tasks to AI systems, human employees are freed to focus on higher-level strategic and creative work. This leads to increased productivity and a more efficient autonomous artificial intelligence.
- Scalability: Agentic AI systems can easily scale to handle increasing volumes of data and tasks. This scalability ensures that businesses can grow without experiencing a corresponding increase in operational complexity.
- Cost Savings: Automating processes with agentic AI reduce labor costs and minimizes errors associated with manual operations. The efficiency gains also lead to significant cost savings in the long run.
- Enhanced Customer Experiences: By leveraging real-time data and adaptive algorithms, AI agents can deliver personalized and timely interactions with customers, improving overall satisfaction and engagement.
Key Considerations for Implementing Agentic AI
While agentic AI offers numerous advantages, enterprises need to proactively address potential challenges to prevent negative outcomes:
- Complexity in Implementation: Integrating agentic AI into existing systems can be complex and challenging. It requires careful planning, customization, and alignment with current workflows to ensure seamless adoption.
- Data Privacy and Security Concerns: Autonomous business intelligence involves handling large volumes of sensitive data. Ensuring data privacy and security is crucial to prevent breaches and misuse of information.
- Bias and Fairness Issues: While agentic AI has the potential to enhance decision-making, it can also unintentionally perpetuate biases present in training data. Implementing rigorous monitoring and correction mechanisms is essential to ensuring fair and unbiased outcomes.
- High Initial Costs: The development and deployment of agentic AI solutions can involve significant upfront costs, including investments in technology, infrastructure, and training. However, these expenses are offset by the long-term gains in efficiency and innovation that agentic AI can bring.
- Dependence on Quality Data: The effectiveness of agentic AI relies heavily on the quality of the data it processes. Robust data collection and management practices are key to minimizing the risks of inaccurate results and enhancing overall performance.
- Ethical and Regulatory Challenges: As AI systems become more autonomous, ethical considerations and regulatory compliance become increasingly important. Ensuring that agentic AI operates within legal and ethical boundaries is essential to maintaining trust and legitimacy.
Approach to Unifying Siloed Data and Ensuring Compliance with AI Co-Workers Built on E42
E42’s sophisticated AI models can autonomously interact with and extract value from vast datasets. E42 employs on-premises Large Language Models (LLMs) that are customized to fit the specific needs and data architectures of enterprises. These LLMs can interact with various data sources within an organization, regardless of the format or location of the data. By using advanced Natural Language Processing (NLP) and machine learning techniques, AI co-workers built on the E42 platform can extract, aggregate, and analyze data from disparate systems. This results in a unified view of enterprise data, breaking down silos and enabling more autonomous business intelligence and informed decision-making while ensuring compliance with industry regulations and data protection standards.
How AI Co-Workers Built on E42 Collaborate to Transform Business Operations
Here’s how AI co-workers built on E42 when deployed across an organization, collaborate seamlessly to optimize and streamline end-to-end enterprise operations across various business functions. Let’s take the AI Marketing Analyst, AI Business Development Executive, and AI Customer Care Executive as examples.
The AI Marketing Analyst gathers and processes intelligent data, which is then utilized by the AI Business Development Executive to engage more effectively with leads, enhancing topline growth. For instance, when a visitor lands on a company’s website, the AI Business Development Executive, informed by insights from the AI Marketing Analyst, can identify the visitor’s buying intent and tailor engagements to their specific interests.
Simultaneously, the AI Customer Care Executive uses data from the AI Marketing Analyst’s social media listening to pinpoint customer issues, particularly those related to competitors. This information is invaluable for the sales team, allowing them to leverage this competitive intelligence for upselling opportunities.
To elevate your enterprise with AI co-workers built for each enterprise function, talk to us today!