Generative AI, with its ability to analyze vast amounts of data and create new, imaginative content across various domains—stands at the forefront of technological innovation. However, this transformative potential is not without its challenges. One of the most critical is the inherent risk of biases in generative AI outputs.
What is bias in generative AI? According to a study, generative AI models can exhibit systematic gender and racial biases, subtly influencing facial expressions and appearances in generated images. This bias is not just confined to the realm of image generation but extends to various applications of AI, including recruitment tools, facial recognition systems, credit scoring, and healthcare diagnostics. For instance, consider the case of a job search platform that was found to offer higher positions more frequently to men of lower qualification than women. While companies may not necessarily hire these men, the model’s biased output could potentially influence the hiring process. Similarly, an algorithm designed to predict the likelihood of a convicted criminal reoffending was questioned for its potential racial and socioeconomic bias.
Understanding the Factors Behind Bias in Generative AI
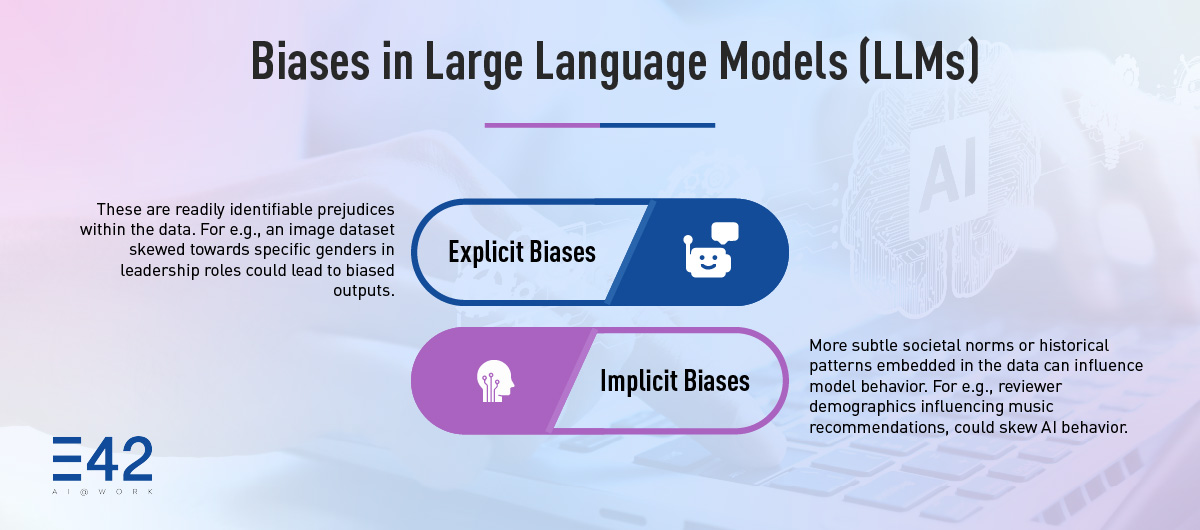
Generative AI models, particularly those based on deep learning techniques such as Generative Adversarial Networks (GANs), including Large Language Models (LLMs) learn to produce new data by analyzing patterns and relationships within large datasets. These models are trained on diverse sources of information, ranging from text and images to numerical data, which serve as the foundation for their creative processes. However, the training data itself may contain biases—both explicit and implicit—that AI systems can inadvertently absorb and perpetuate. Therefore, understanding and addressing bias in LLMs is a crucial aspect of ethical AI development.
Impact of Biased Generative AI
The ramifications of biases in generative AI outputs can influence various facets of society and daily life. A generative AI bias can be likened to hallucinations—where the AI may invent content not present in the training data, potentially leading to factual inconsistencies and harmful outputs. Moreover, biased AI-generated content can prompt toxicity, perpetuating societal prejudices and misinformation. Here’s how these biases impact society:
- Perpetuating Discrimination: Biased AI algorithms can reinforce existing societal prejudices, leading to discriminatory outcomes in critical areas such as hiring practices, loan approvals, and law enforcement technologies like facial recognition.
- Spreading Misinformation: AI-generated content, including deepfakes and fake news articles, can proliferate biases present in the training data. This misinformation can mislead the public and erode trust in reliable sources of information, exacerbating social divisions and undermining democratic processes.
- Eroding Trust in Technology: When users encounter biased outputs from AI systems, whether in personal interactions or through digital platforms, it can diminish confidence in AI technologies as impartial and objective tools. This distrust can impede the widespread adoption of AI solutions and limit their potential benefits across industries.
Mitigating Bias: Strategies and Approaches
Addressing biases in generative AI demands a holistic approach that spans the entire lifecycle of development—from initial data collection and model training to deployment and ongoing monitoring.
It begins with curating diverse datasets, a critical step that ensures training datasets reflect diverse perspectives and demographics. This involves not only actively seeking data from underrepresented groups but also employing techniques to balance any skewness in the data distribution. By fostering inclusivity in the data used to train AI models, we can mitigate the risk of biases that may otherwise be perpetuated in AI-generated outputs.
This process is complemented by the implementation of debiasing techniques. These advanced algorithms and methodologies, such as data augmentation, fairness-aware model training, and post-processing adjustments, play a pivotal role in minimizing biases within datasets and AI models. They are designed to detect and mitigate biases at various stages of AI development, ensuring that AI systems produce outputs that are fair and unbiased.
Incorporating human-in-the-loop systems is another essential component of this holistic approach. Human reviewers provide crucial oversight and feedback throughout the AI development process. They are instrumental in identifying potential biases in AI-generated outputs that algorithms may overlook. Their insights and ethical judgment contribute to refining AI models and ensuring that decisions made by AI systems align with ethical standards and societal values.
Subsequently, algorithmic transparency enhances the stakeholders’ understanding of AI decision-making processes. Transparent AI models allow users to comprehend how decisions are reached and identify potential biases early on. By fostering transparency, we promote accountability and enable timely intervention to mitigate biases. This not only fosters trust and confidence in AI technologies among users and stakeholders but also ensures that our AI systems are both effective and equitable.
Ethical Guidelines and Responsible AI Practices
What are some ethical considerations when using generative AI? Before answering that question, here’s a report by the Capgemini Research Institute which reveals that about 62% of consumers have more trust in companies that they believe use AI ethically. This highlights the importance of ethical considerations and responsible practices in AI and navigating the ethical implications of generative AI bias requires proactive measures to mitigate biases and uphold gen AI ethics:
- Factual Accuracy: Ensuring that AI-generated content aligns closely with verified information is crucial to prevent the dissemination of misinformation. Techniques like Retrieval Augmented Generation (RAG) play a pivotal role here, as they enhance the reliability of outputs by grounding them in factual accuracy. By leveraging RAG and similar methodologies, AI systems can reduce the risk of biased or misleading information reaching the public, thereby upholding integrity in information dissemination.
- Toxicity Mitigation: Addressing toxicity in AI-generated content involves implementing robust measures such as context-aware filtering and content moderation. These techniques enable AI models to recognize and suppress harmful or offensive outputs effectively. By proactively filtering content that may provoke negative reactions or propagate harmful stereotypes, AI systems contribute to maintaining a safe and respectful digital environment for users.
- Validation Protocols: Establishing stringent validation processes is essential to verify the authenticity and fairness of AI-generated outputs. Techniques like 2-way and n-way matching against established criteria ensure that AI systems operate ethically and responsibly. By validating outputs rigorously, developers can mitigate the risk of biased outcomes, thereby fostering trust among users and stakeholders in the reliability and ethical integrity of AI technologies.
The Importance of Fairness Metrics
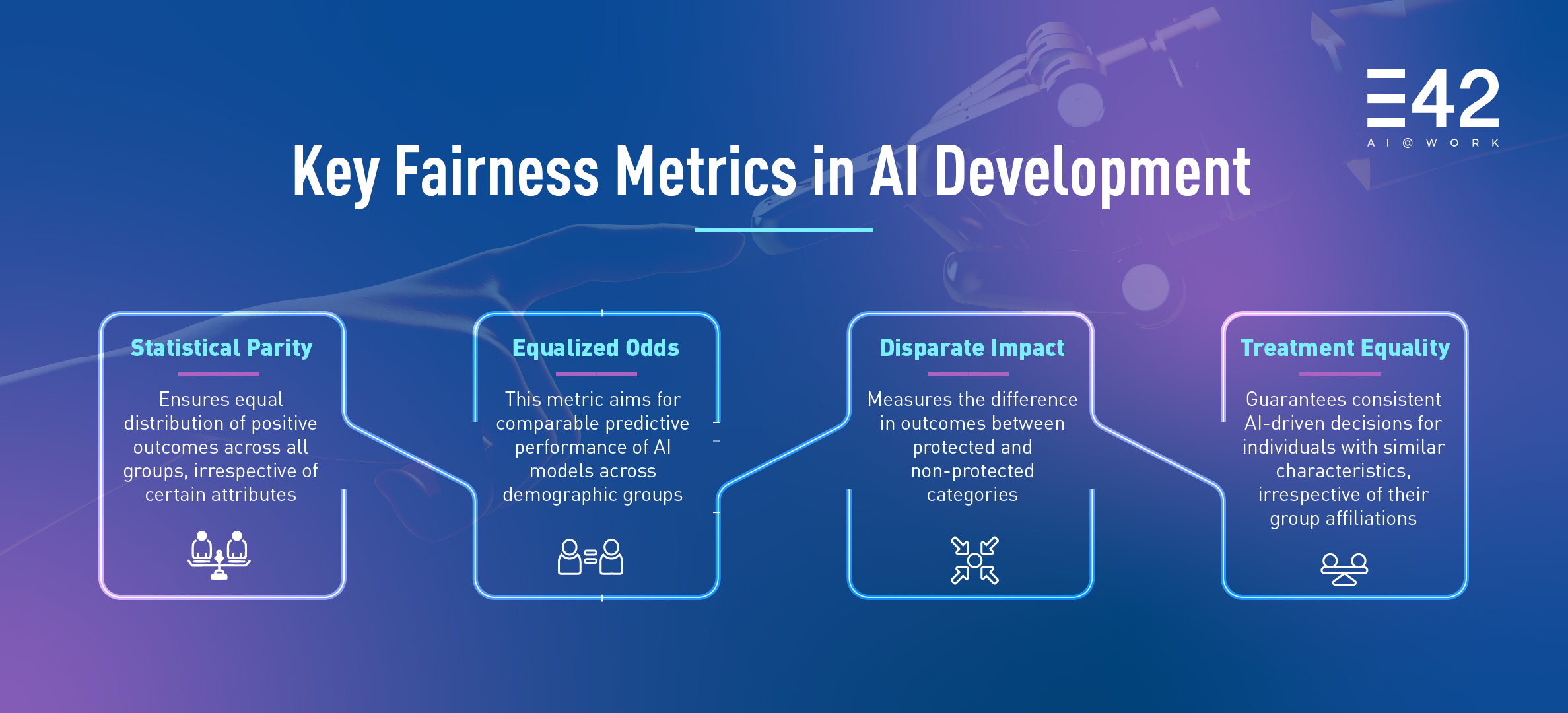
Fairness metrics are crucial quantitative measures used in the AI development lifecycle to evaluate and mitigate biases in generative AI, aligning with gen AI ethics and equitable operation across diverse user demographics. Here’s an exploration of key fairness metrics:
- Statistical Parity: This metric compares the distribution of outcomes, such as loan approvals or job offers, across different demographic groups. It ensures that the proportion of positive outcomes is similar across all groups, irrespective of sensitive attributes like race or gender.
- Equalized Odds: Focuses on the predictive performance of AI models across demographic groups. It aims to achieve comparable true positive rates (correct predictions for positive cases) and false positive rates (incorrect predictions for negative cases) for all groups.
- Disparate Impact: Measures whether there are statistically significant differences in outcomes between protected and non-protected groups based on sensitive attributes like race or gender. For instance, in hiring decisions, disparate impact analysis evaluates whether there is an imbalance in selection rates between male and female applicants.
- Treatment Equality: Assesses whether individuals with similar characteristics receive comparable predictions or decisions from the AI model, regardless of their demographic attributes. Treatment equality ensures consistency in AI-driven decisions, promoting fairness and transparency in how outcomes are determined across different groups.
The Road Ahead: Building a Fairer Future with Generative AI
Generative AI stands at a pivotal juncture, brimming with potential to reshape various aspects of our world. However, to ensure its responsible integration, addressing the challenge of bias is paramount. This necessitates a multi-pronged approach encompassing diverse data curation, advanced debiasing techniques, and unwavering commitment to ethical considerations.
By fostering collaboration between developers, data scientists, policymakers, and the public, we can establish robust frameworks that prioritize fairness, transparency, and accountability in generative AI development and deployment and in turn mitigate biases in LLMs. Continuous investment in research and development, coupled with the ongoing refinement of training methodologies and validation protocols, will further empower AI systems to deliver unbiased and reliable outputs.
To leverage gen AI for your enterprise operations with E42, get in touch with us today!