The world of artificial intelligence is brimming with excitement surrounding generative AI models. These advanced machine learning tools hold the remarkable ability to create realistic text, captivating visuals, and even innovative product designs. But what if you could push them even further? That’s what brings us to the question, how to train generative AI models to optimize your AI strategy? Imagine transforming these versatile yet generic tools into bespoke instruments designed specifically for your unique needs. This is the power of custom training, where you can unlock the full potential of generative AI and tailor it to become a laser-focused partner for your specific goals.
Pre-Trained Powerhouses: A Foundation with Limitations
Large Language Models (LLMs) in generative AI play a pivotal role. These models are trained on vast amounts of text data, enabling them to generate human-like text based on the input they receive. However, as powerful as these LLMs in generative AI are, they share the same limitations as other pre-trained models. While they can handle a broad range of tasks with remarkable versatility, they may lack the nuanced understanding required to navigate the intricacies of specific industries or the unique writing style necessitated by certain tasks.
For instance, an LLM might generate a technically accurate product description, but it wouldn’t capture the level of technical precision demanded by a scientific paper. Similarly, crafting marketing copy for a legal firm using an LLM necessitates a distinct tone and level of formality compared to a children’s clothing brand.
While generative AI holds immense potential, here are some more reasons why enterprises should exercise caution when using pre-trained LLMs:
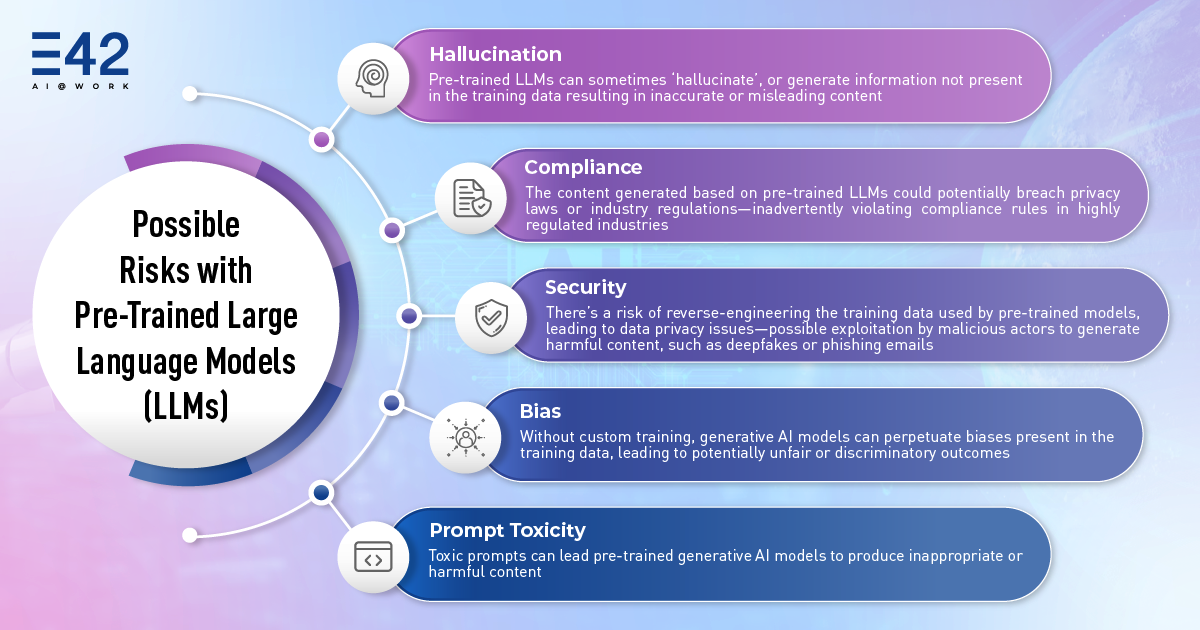
- Hallucination: While pre-trained Language Models (LLMs) offer impressive capabilities, they can sometimes exhibit what’s known as ‘hallucination,’ generating information not present in the training data. This may lead to inaccurate or misleading content, undermining the reliability of generated text.
- Compliance: Leveraging pre-trained LLMs to generate content raises concerns regarding compliance with privacy laws and industry regulations. In regulated sectors, such as healthcare or finance, there’s a heightened risk of inadvertently violating compliance rules. Content generated by these models may unknowingly breach sensitive data privacy regulations, posing legal and reputational risks for organizations.
- Security: One significant security concern associated with pre-trained models is the potential for reverse-engineering the training data. Malicious actors could exploit this vulnerability to access sensitive information, leading to severe data privacy breaches. Such breaches could enable the creation of harmful content, including deepfakes or phishing emails, posing significant threats to individuals and organizations alike.
- Bias: Without custom training, generative AI models may inadvertently perpetuate biases present in the training data. This can result in the generation of content that reflects and reinforces existing societal biases, leading to potentially unfair or discriminatory outcomes. Addressing bias in generative AI models is crucial for ensuring equitable and ethical use across various applications and domains.
- Prompt Toxicity: Another challenge arises from the potential toxicity of prompts used to interact with pre-trained generative AI models. Toxic prompts can lead these models to produce inappropriate or harmful content, posing risks to users and undermining trust in AI-driven systems. Careful consideration of prompt design and monitoring mechanisms is essential to mitigate the risk of prompt-induced toxicity in generated content.
This is where the power of custom training a generative AI model shines. By refining the LLM’s comprehension and tailoring its outputs to specific requirements, one can overcome these limitations. Custom training allows the generative AI model to better understand and generate content that aligns more closely with the specific needs of the task at hand.
Custom Training: Honing the Generative Edge
Envision custom training as the act of equipping that master chef with a treasure trove of specialized ingredients—industry reports, company data, successful marketing campaigns tailored to your specific niche. By feeding these unique elements to the generative model, you essentially educate it in the language of your field. This focused training empowers the model to grasp the complexities of your domain, the preferred writing styles, and the data formats you typically encounter.
The advantages are undeniable. A meticulously custom-trained model transforms into a master of its domain, generating outputs that are not only demonstrably more creative but also demonstrably more accurate and effective. Imagine the efficiency of having an AI tool that can generate product descriptions perfectly aligned with your brand voice, or a model that creates legal documents tailored to your firm’s established practices.
How to Train Generative AI Model
Let’s delve into the process of constructing and training a generative AI model in a more detailed and structured manner:
- Defining Objectives: The first step is for one to clearly define what they want their AI model to achieve. This could range from generating product design ideas that align with their brand’s aesthetic to crafting personalized marketing campaigns that resonate with their target audience.
- Data Selection: The next step is for them to select the right data for training their model. The quality and relevance of their data are crucial. Industry-specific datasets are often the best choice. For example, if they’re in the legal field, legal documents and case studies would be invaluable. A marketing team might leverage customer data and successful past marketing campaigns.
- Data Preparation: Once they’ve selected their data, it needs to be prepared for training. This involves cleaning the data (removing irrelevant information, dealing with missing values, etc.), and transforming it into a format that can be understood by the model. This step also includes deciding which data should be used for training, validation, and testing.
- Model Selection and Training: The next step is for them to choose the right model architecture for their task and feed their prepared data into it. The model learns by analyzing the patterns and relationships in their data. This is where complex algorithms and neural networks come into play. However, the core concept is simple: the model learns to generate outputs similar to their training data.
- Evaluation and Calibration: After the model has been trained, it’s important for them to evaluate its performance and calibrate it as necessary. This might involve adjusting the model’s parameters, adding more data, or even changing the model architecture.
- Deployment: Once the model is performing well, it can be deployed in their desired environment. This could be on-premises, in the cloud, or even on edge devices.
- Monitoring and Maintenance: After deployment, the model should be regularly monitored to ensure it continues to perform well as new data comes in. It may need to be retrained or adjusted over time.
Remember, the more focused and relevant their data, the more adept their custom model will become at replicating the desired outputs. This process is iterative and may require several rounds of training and adjustment to achieve the best results.
Advantages of a Custom-Trained Gen AI Model
The fruits of your meticulous labor manifest in the form of a custom-trained model that boasts a range of significant advantages over its pre-trained counterparts. Here’s a taste of what you can expect:
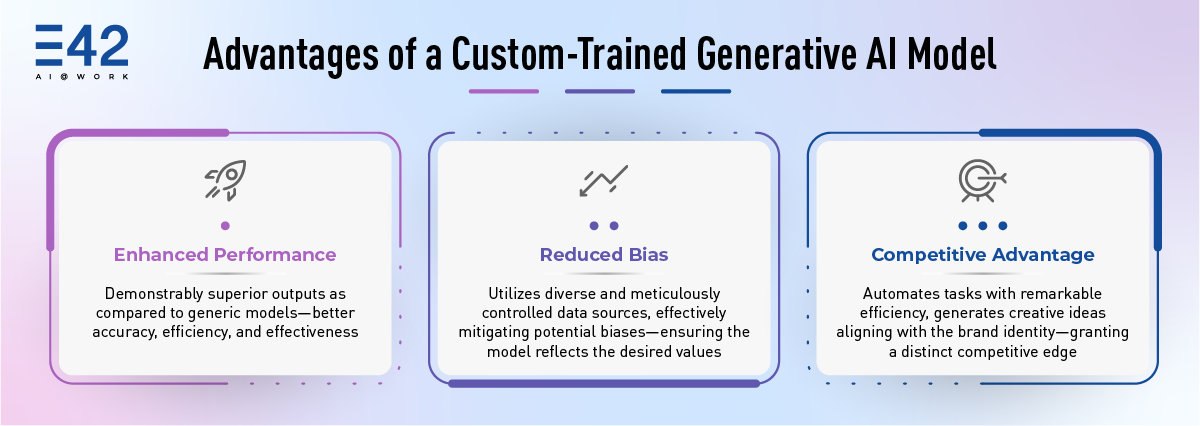
- Enhanced Performance: Accuracy, efficiency, and effectiveness—these are the hallmarks of a meticulously custom-trained model. It possesses a profound understanding of your specific requirements and delivers outputs demonstrably superior to generic models.
- Reduced Bias: Pre-trained models can inherit biases from the vast datasets they are trained on. Custom training empowers you to utilize diverse and meticulously controlled data sources, effectively mitigating potential biases and ensuring your model reflects your desired values.
- Competitive Advantage: In today’s data-driven world, a custom-trained model can be a game-changer. It equips you with a powerful tool that can automate tasks with remarkable efficiency, generate creative ideas that seamlessly align with your brand identity, and ultimately grant you a distinct edge within your industry.
Conclusion
In conclusion, tailoring a generative AI model to meet specific needs is a meticulous and iterative process. It begins with a clear definition of objectives (what do enterprises need to consider with generative AI), followed by careful data selection and preparation. The chosen model is then trained and calibrated using this data, with the aim of generating outputs that align closely with the desired outcomes. Once satisfactory performance is achieved, the model is deployed and continuously monitored for optimal results. Remember, the key to success lies in the relevance and quality of the data used for training. With the right approach and resources, one can harness the power of generative AI to drive innovation and efficiency in their specific field.
Leveraging Generative AI for Enterprise Automation with E42
LLMs trained on generic data have a general linguistic understanding that seldom allows them to be used as-is. For many businesses, the valuable uses of LLMs require that the model be taught new behavior that is relevant to a particular application. E42’s roadmap covers leveraging LLMs for business applications and conditioning the LLMs for domain-specific behavior. From AI-driven responses and FAQ generation to text summarization, code generation, content creation, and more, E42’s LLM capabilities empower businesses to enhance efficiency, streamline operations, and unlock new possibilities in document management.
To know more about generative AI and make the most of out of the technology for your business, get in touch with us today!