Welcome to a world where artificial intelligence (AI) not only mirrors human thought processes but elevates them to new heights, introducing a level of creativity and dynamism comparable to the human mind. In this realm of possibilities, Large Language Models (LLMs) emerge as the architects, shaping a digital landscape where AI doesn’t just analyze data but innovates, creates, and imagines across diverse domains.
Here’s what this article covers:
What is Generative AI?
Generative AI lies at the core of autonomous content creation through sophisticated algorithms. Unlike traditional AI systems that primarily process and analyze data, generative AI takes it a step further by autonomously creating content. This creative autonomy is achieved through complex algorithms that enable the system to generate new, contextually relevant content, transcending predefined patterns.
The journey of generative AI commenced with rudimentary approaches and rule-based systems, setting the stage for advancements. Neural networks marked a pivotal shift, and LLM capabilities have further propelled this evolution, introducing a paradigm shift in content generation.
What are Large Language Models?
Large Language Models are advanced AI models that specialize in understanding and generating human-like text. They are trained in diverse datasets, enabling them to comprehend and generate language in a contextually relevant and coherent manner. LLMs in generative AI are revolutionizing the field of natural language processing, finding applications across various industries, and enhancing human creativity.
LLMs stand out from their predecessors due to a key differentiator—context. Take GPT-4, for instance, capable of processing up to 1500 words of context. This extended context allows for a more nuanced understanding of the user’s request. Moreover, the cutting-edge language modeling tools are now multimodal. This means they can adeptly handle various input formats, whether it’s text, video, or images. Now, let’s delve deeper into LLMs and their capabilities:
- Understanding Language: LLMs are trained on vast amounts of data, which enables them to understand the nuances and intricacies of language. They can comprehend context, interpret meaning, and generate responses that are relevant and coherent.
- Generating Human-like Text: Unlike traditional language models that focus on generating text based on statistical patterns, LLMs can understand and generate language in a way that’s much closer to how humans do. LLMs in generative AI understand context, infer meaning, and generate responses or content that’s relevant to the given input.
- Parameter-Rich Architectures: LLMs surpass conventional models with an extensive number of parameters, offering a nuanced understanding of language intricacies and enabling large-scale text generation.
- Diversification of Input Data: LLM capabilities transcend textual boundaries, incorporating multimodal capabilities that integrate diverse data types, such as images and text, broadening their scope and capabilities.
The Significance of On-Premises Large Language Models
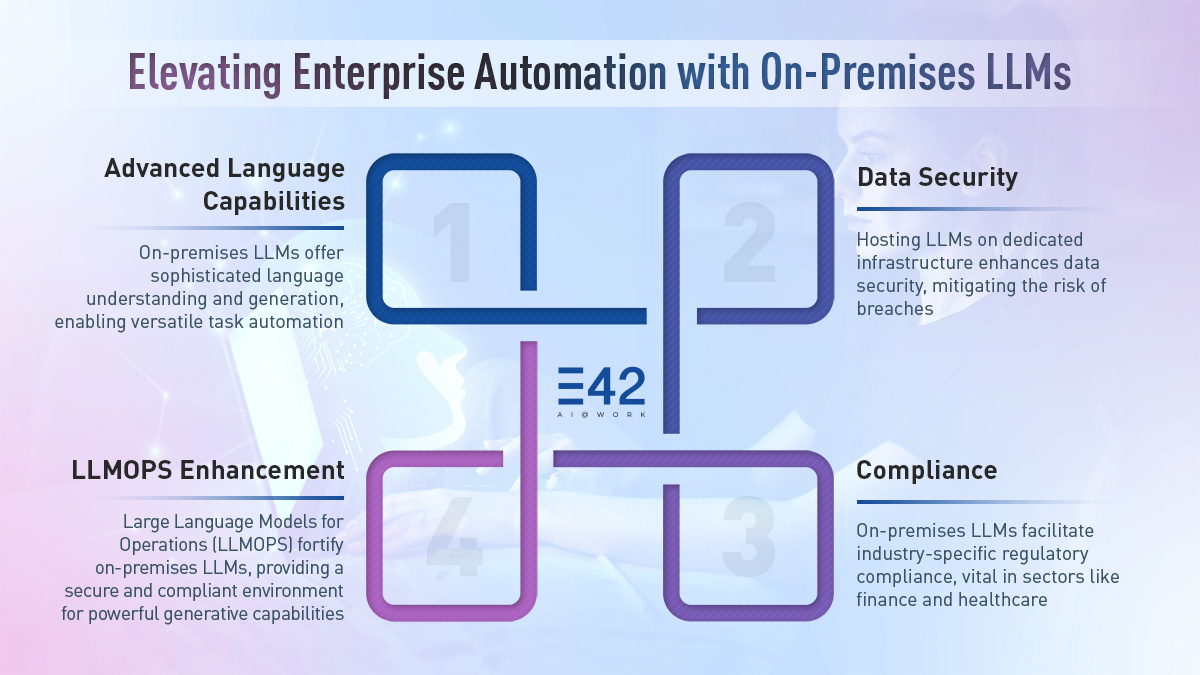
In the age of AI-led digital transformation, organizations are increasingly leveraging advanced technologies to streamline their operations, and one such innovation taking the spotlight is on-premises (on-prem) Large Language Models. These models usher in a new era of advanced language capabilities, empowering organizations to automate an extensive array of tasks seamlessly. What sets on-prem LLMs apart is their unparalleled commitment to data security. By hosting these models on their own infrastructure, organizations fortify their defenses against potential data breaches, ensuring a robust shield for sensitive information.
Moreover, in sectors like finance and healthcare, on-prem LLMs serve as a compliance enabler, allowing organizations to navigate the intricate web of industry-specific regulations with ease. They align seamlessly with stringent standards and ensure that data practices adhere to the highest norms.
A standout addition to this paradigm is the advent of Large Language Models for Operations (LLMOPS). These frameworks act as force multipliers, enriching on-prem LLMs with additional capabilities— transforming them into formidable assets for organizations. LLMOPS not only elevate generative capabilities but also establish a secure and compliant environment for organizations to harness the full potential of LLMs. In essence, the integration of on-prem LLMs and LLMOPS marks a transformative journey for organizations, opening new horizons of automation, security, and compliance.
With the complexities of AI-driven automation, deploying on-prem LLMs, backed by enhanced security and enriched by LLMOPS, empowers the full utilization of advanced language models, making the most of their potential in the enterprise ecosystem. To optimize such a model for business requirements, precision tuning with proprietary data is crucial, especially in on-premises or private cloud deployments.
This tailored approach empowers the model to elevate complex workflows and refine business processes. Choosing this method significantly enhances the potential benefits of an LLM compared to alternative adoption strategies. A customized model, influenced by specific training data, excels in diverse tasks such as sentiment analysis, automated content creation, intelligent document processing, and more.
Real-World Applications of LLMs in Generative AI
LLMs transcend industry boundaries, finding applications in a myriad of sectors. In legal practices, they streamline documentation creation, minimizing errors and enhancing efficiency. The financial sector benefits from their data analysis capabilities, aiding in market predictions and optimizing reporting processes. Beyond law and finance, LLMs make substantial contributions to healthcare by analyzing extensive medical literature for research purposes. In marketing, LLMs excel at optimizing advertising strategies, ensuring targeted messaging, and enhancing creative collaboration.
Moreover, LLMs show promise in revolutionizing customer support by crafting personalized responses and handling queries with a human touch. In the educational realm, they facilitate automated content generation, aiding in the creation of diverse learning materials. This adaptability makes them versatile assets capable of reshaping various aspects of how we work and interact, and not merely tools for specific industries. As technology continues to evolve, the range of applications for LLMs in generative AI is bound to expand, further solidifying their position as transformative agents in the broader landscape of artificial intelligence.
Exploring Future Trends in Generative AI
As we delve into the future, the landscape of generative AI with LLMs is poised for continual evolution. Anticipated trends include enhanced multimodal capabilities, increased fine-tuning for specific industries, and breakthroughs in mitigating biases.
- Industry-Specific Fine-Tuning: The future of LLM capabilities lie in their adaptability. There is an increasing focus on fine-tuning these models for specific industries to cater to their unique requirements and nuances. This involves customizing models to better serve sectors like healthcare, finance, legal, and others, ensuring more accurate and industry-relevant content generation.
- Bias Mitigation Breakthroughs: As we move forward, we anticipate significant breakthroughs in addressing and mitigating biases within LLMs. This involves the development of advanced algorithms and methodologies to identify and rectify biases in training data. The goal is to ensure fair and unbiased content generation, promoting the ethical and responsible use of generative AI.
- Quantum Leap in Model Size and Complexity: The evolution of LLMs is expected to take a quantum leap—future models are predicted to handle even more parameters and intricate architectures. This will involve the exploration of models that can capture an unprecedented level of detail and intricacy, pushing the boundaries of what LLMs can achieve in terms of content generation.
Conclusion
The fusion of generative AI with LLMs unfolds a panorama of sophisticated output, highlighting the vast potential for AI to redefine the boundaries of creativity. As the field evolves, the role of LLMs in generative AI stands as a testament to the symbiotic relationship between technology and creative expression. The meticulous approach to ethical considerations and human oversight ensures responsible and innovative advancements in content generation.
Looking ahead, the journey of generative AI with LLMs opens doors to a synergy where human ingenuity collaborates with machine intelligence to create fantastic outcomes. The ongoing exploration of this synergy promises a conscientious integration that respects ethical boundaries and human values. The future of generative AI, marked by the continuous refinement and augmentation of LLMs, holds the potential to elevate creativity and innovation to unprecedented heights, shaping a landscape where the boundaries between human and machine creativity become increasingly blurred.
Make your Enterprise Intelligent with E42
E42 is a no-code Cognitive Process Automation (CPA) platform to create multifunctional AI co-workers that automate enterprise functions across verticals and domains ranging from automobiles and BFSI to telecom, manufacturing, and more. By maximizing efficiency and scalability, and minimizing the human workload, E42 is disrupting the enterprise automation space while delivering a hassle-free user experience. To kickstart your enterprise automation journey, write to us at interact@e42.ai!